Quantum processors and sensors promise to outperform their classical counterparts. Still, they require classical control signals, which affect their operation in a highly nontrivial way. An example: electrical voltages controlling a photonic chip or a superconducting circuit.
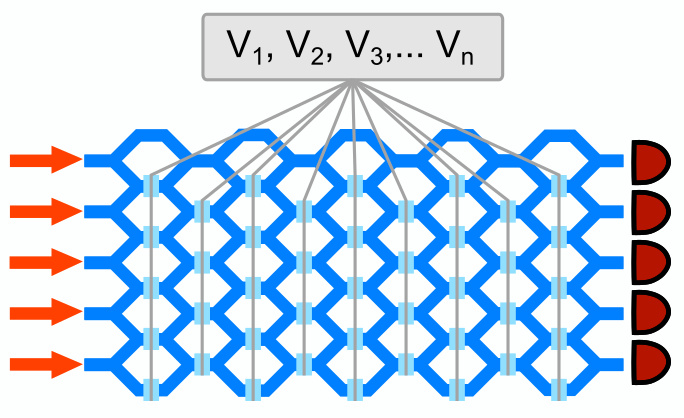
We developed a ML approach for finding the optimum values of the control signals. It uses a pair of cooperating artificial neural networks learning the optimal quantum device operation independently of a number and type of classical control signals.
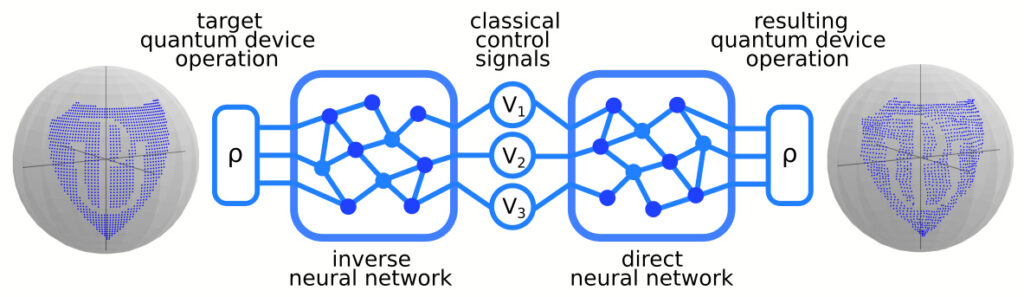
The trained networks allow for bidirectional control — predicting the quantum device operation from control signals and predicting the optimal control signals for a target operation of the device. Publication: 10.1103/PhysRevApplied.17.054042, preprint: https://arxiv.org/abs/2109.12436, data&code: https://github.com/VasinkaD/NeuralLC.
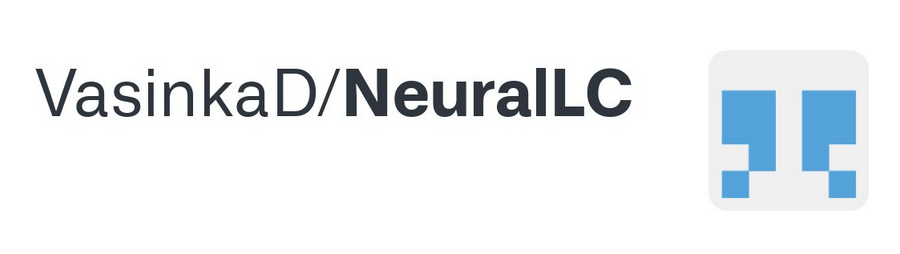
We demonstrated the approach by controlling light polarization with liquid crystals. Liquid crystals can quickly modify the polarization, but their notoriously complex behavior eludes accurate description. Links to publications: 10.1364/OE.388675, 10.1103/PhysRevApplied.17.054042.
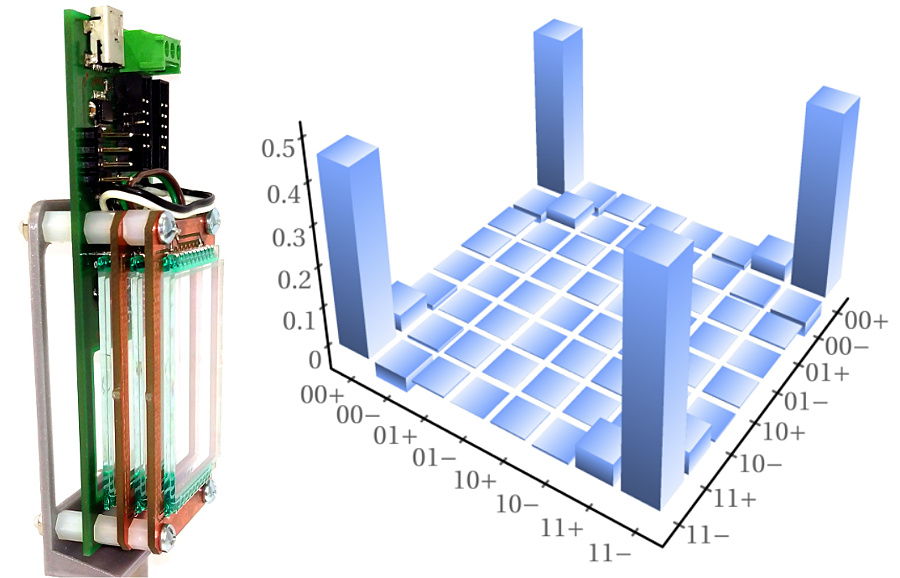